Reducing the problem of face recognition to an average February 5, 2008
Posted by Johan in Applied, Cognition, Face Perception, Theory.trackback
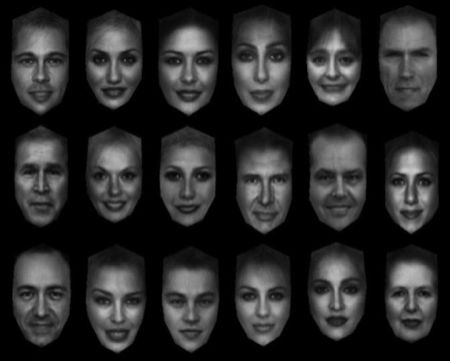
Although computer software is now adept at face detection – Google’s image search does it, and so does you camera if you bought it within the past year – the problem of recognising a face as belonging to a specific individual has proved a hard nut to crack.
Essentially, this is a problem of classification. A model for this process should be able to sort images of three persons into three separate categories. This is remarkably difficult to do. If you look at the sheer physical differences between images of the same person, they easily outnumber the differences between images of different persons, taken from the same angle under the same lighting conditions. In other words, the bulk of the physical variability between different face images is uninformative, as far as face recognition is concerned. Thus, this remains an area where humans effortlessly outperform any of the currently-available face recognition models.
Recent work by Mark Burton at the Glasgow Face Recognition Group suggests a solution by which computer models can achieve human-like performance at face recognition. By implication, such a model may also offer a plausible mechanism for how humans perform this task. The model that Burton et al (2005) proposed is best explained by this figure, which outlines the necessary processing steps:
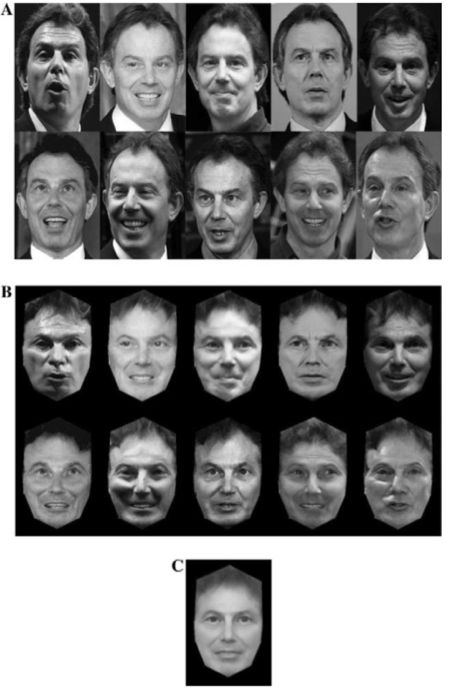
For each face that the model is to learn, a number of example images are collected (as shown in A). These images are morphed to a standard shape (B), which makes it possible to carry out pixel-by-pixel averaging to create a composite (C). This composite is then used by the model to attempt to recognise a new set of images of the person.
This may sound relatively straight-forward, but the idea is novel. Most face recognition models that work with photographs use an exemplar-based algorithm, where the model stores each of the images it is shown. Such models do improve as more faces are added (since there are more exemplars that might possibly match), but not as much as an averaging model does as more pictures are added to the average (Burton et al, 2005). Furthermore, when noise is added in the form of greater variations in lighting, the exemplar model breaks down rapidly while the averaging model is largely unaffected.
Why is this model so effective? The averaging process appears to remove variability that is not relevant to personal identity (such as differences in lighting and shading, changes in hair style), while preserving information that is informative for recognition (eyebrows, eyes, nose, mouth, perhaps skin texture). The figure at the top of this post highlights this (from Burton et al, 2005). The pictures are shape-free averages, created from 20 exemplar pictures of each celebrity. To the extent that hair is present, it is usually blurry. But the pictures are eminently recognisable, even though you have in fact never seen any of these particular images before (since they are composites). Indeed, Burton et al (2005) showed that participants were faster to recognise these averages than they were at recognising the individual exemplar pictures.
In the latest issue of Science, Jenkins and Burton (2008) presented an unusual demonstration of the capabilities of this model. They pitted their model against one of the dominant commercial face-recognition systems (FaceVACS). The commercial model has been implemented at MyHeritage, a website that matches pictures you submit to a database of celebrities.
Jenkins and Burton (2008) took advantage of this by feeding the website a number of images from the Burton lab’s own celebrity face database. Note that the website is all about matching your face to a celebrity, so if an image of Bill Clinton from the Burton database is given as input, you would expect the face recognition algorithm to find a strong resemblance to the Bill Clinton images stored by MyHeritage. Overall, performance was unimpressive – 20 different images of 25 male celebrities were used, and the commercial face algorithm matched only 54% of these images to the correct person. This highlights how computationally difficult face recognition is.
In order to see how averaging might affect the model’s performance, Jenkins and Burton (2008) took the same 20 images and created a shape-free average for each celebrity. Each average was then fed into the model.
This raised the hit rate from 54% to 100%.
The model that Burton is advocating is really one where individual face images are recognised with reference to a stored average. This finding is essentially the converse – the commercial model, which attempts to store information about each exemplar, is used to identify an average. But there is no reason why it wouldn’t work the other way around.
This demonstration suggests that as far as computer science is concerned, the problem of face recognition may be within our grasp. There are a few remaining kinks before we all have to pose for 20 passport pictures instead of one, however: the model only works if each exemplar is transformed, as shown in the figure above. As I understand it, this process cannot be automated at present.
While we’re on the computer science side I think it is also worth mentioning that there may be some ethical implications to automatic face recognition, especially in a country with one CCTV camera for every 5 inhabitants (according to Wikipedia). I have always dismissed the typical Big Brother concerns with the practical issue of how anyone would have time to actually watch the footage. If, however, automatic face recognition becomes common-place, you had better hope that your government remains (relatively) benevolent, because there will be no place to hide.
Turning to psychology, the assertion by Burton et al is that this model also represents to some extent what the human face recognition system is doing. This sounds good until you realise that face recognition is not hugely affected by changes in viewing position – you can recognise a face from straight on, in profile, or somewhere in between. This model can’t do that (hence the generation of a shape-free average), so if the human system works this way, it must either transform a profile image to a portrait image in order to compare it to a single, portrait average, or it must store a number of averages for different orientations, which leads to some bizarre predictions (for example, you should have an easier time recognising the guy who sits next to you in lecture from a profile image, because that’s how you have usually viewed him).
That being said, this model offers an extremely elegant account of how face recognition might occur – read the technical description of FaceVACS to get a taste for how intensely complex most conventional face recognition models are (and by implication, how complex the human face recognition system is thought to be). The Burton model has a few things left to explain, but it is eminently parsimonious compared to previous efforts.
References
Burton, A.M., Jenkins, R., Hancock, P.J.B., & White, D. (2005). Robust representations for face recognition: The power of averages. Cognitive Psychology, 51, 256-284.
Jenkins, R., Burton, A.M. (2008). 100% Accuracy in Automatic Face Recognition. Science, 319, 435. DOI: 10.1126/science.1149656
[…] Would you mind if we classify your face? (Johan at Phineas Gage Fan […]
sleep apnea death causes in 2015
Reducing the problem of face recognition to an average | The Phineas Gage Fan Club